Artificial Intelligence (AI) is transforming food quality analysis in the agri-food sector, introducing innovative solutions to enhance accuracy, consistency, and efficiency. Common AI applications in this field include image recognition, spectral analysis, and machine learning-based predictions. These technologies are revolutionising how food quality is assessed, moving away from traditional, subjective methods to more objective, data-driven approaches.
Image Recognition and Spectra Analysis
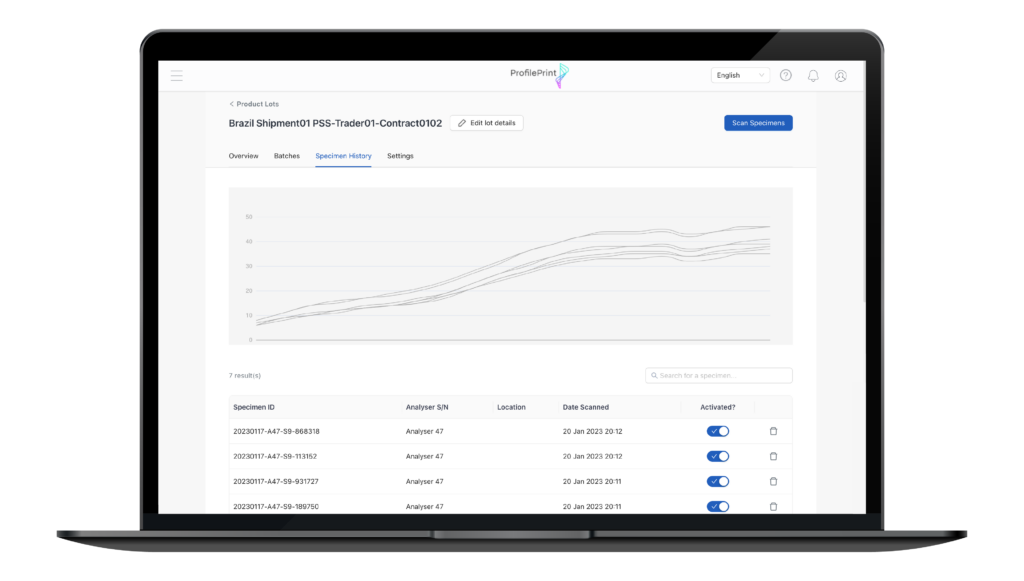
One prominent application of AI in food quality analysis is image recognition, which is used to identify and classify food products based on their visual attributes. For example, AI algorithms can detect visual defects in fruits and vegetables, ensuring only high-quality produce reaches consumers. Spectral analysis, another significant application, involves using AI to interpret data from spectroscopy devices that capture the molecular composition of food samples. This method provides detailed insights into the quality and authenticity of ingredients, enabling precise assessments that are difficult to achieve through conventional techniques.
Challenges of AI in Food Quality Analysis
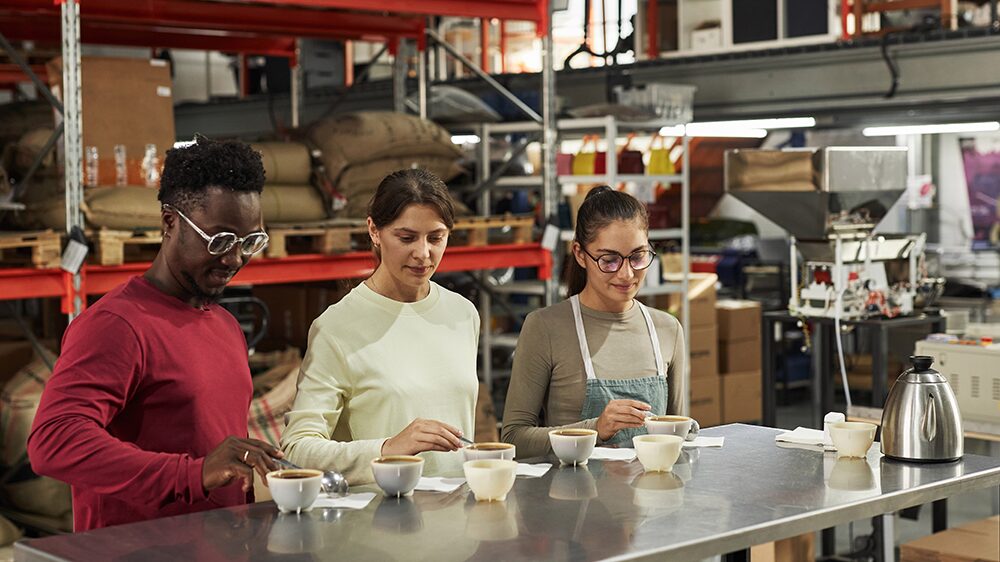
However, applying AI in food quality analysis comes with challenges. One major consideration is the need for extensive and high-quality training data to ensure accurate predictions. As food quality assessments are often based on taste and smell, these can lead to large variability in the data, which would easily confuse an AI model. Furthermore, the problem of data drift is particularly apparent in food quality, as food products can change subtly from season to season, or due to variations in storage or transport conditions. These may lead to a scenario where the taste and smell of the food remain unaffected to the taster, but the underlying chemical makeup could have significantly changed, causing models to grossly mispredict.
Unlock Sensory Attributes through Digital Food Fingerprinting
ProfilePrint is overcoming these challenges by leveraging advanced machine learning techniques and robust data processing methods. Whereas our data collection is based on spectrometric principles, our proprietary technology lies in the ability to process digital fingerprint data to remove noise and account for various sources of variability, as well as exploiting a combinatorial approach towards model building.

With our technology, ProfilePrint has been able to model sensory attributes like bitterness, mouthfeel, and umami to a reasonably accurate extent. Additionally, ProfilePrint continues to develop its technology to procure even more complete digital fingerprints of food products, which will enhance its ability to provide rapid and consistent predictions to help inform food quality assessments.
Beyond Challenges: AI Users in a New Era of Food Quality Analysis
In conclusion, AI is significantly enhancing food quality analysis by providing more precise, objective, and efficient methods. Despite challenges, companies like ProfilePrint are successfully integrating AI to transform the agri-food industry, ensuring better quality control and product development.