Many agricultural products, such as coffee, soybeans, and corn, often have random flaws or imperfections. While visual defects due to mould growth, insect damage, and foreign matter for example are sortable manually, non-visual defects such as over-fermentation, early mould growth and overheated grains are only detectable by sensory evaluation or further processing, which is either cumbersome or would already require committing to the material’s processing.
Recognising the impact on the food and agri industries, ProfilePrint has developed a novel AI-powered solution that is able to sensitively detect non-visual defects. We have demonstrated this firstly in coffee, where quantitation of non-visual defects such as ‘phenolic’ and ‘Rio’ are of great interest as they affect pricing and market targeting.
Existing Methodology and its Challenges
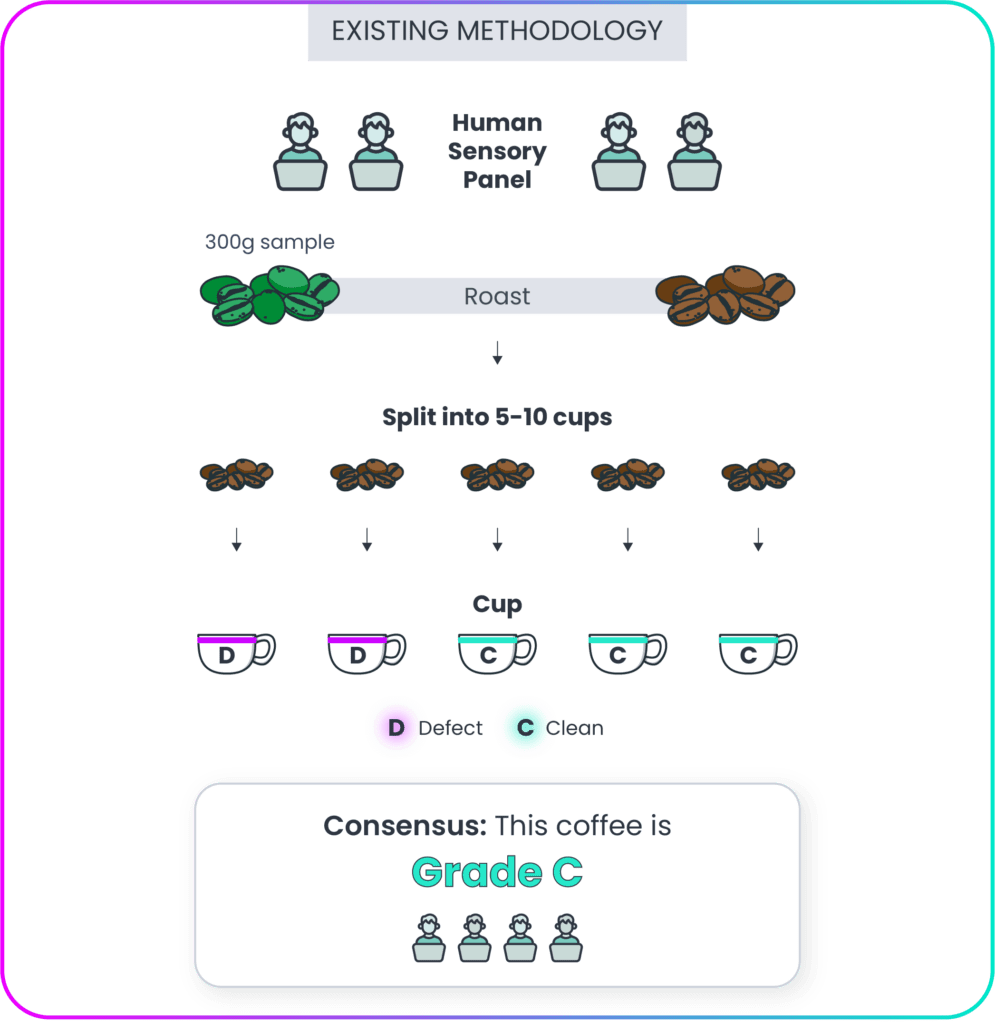
Currently, the coffee industry relies heavily on traditional cupping methods to identify non-visual defects. Many defects, such as phenolic, slight fermentation or early-stage mould, cannot be visually detected, but can significantly affect its quality and taste. The natural variability in coffee beans further complicates the challenge.
Human sensory evaluation is onerous, subjective, and prone to inconsistencies. Furthermore, the cupping process, which involves roasting to brewing coffee beans, is time-consuming and resource-intensive, hindering efficiency and potentially leading to quality control challenges. As some of these defects have a complex flavour component and are also sporadic in nature, the use of conventional analytical chemistry methods has also not led to any significant breakthrough.
Overcoming Non-Visual Defect Detection with ProfilePrint’s AI
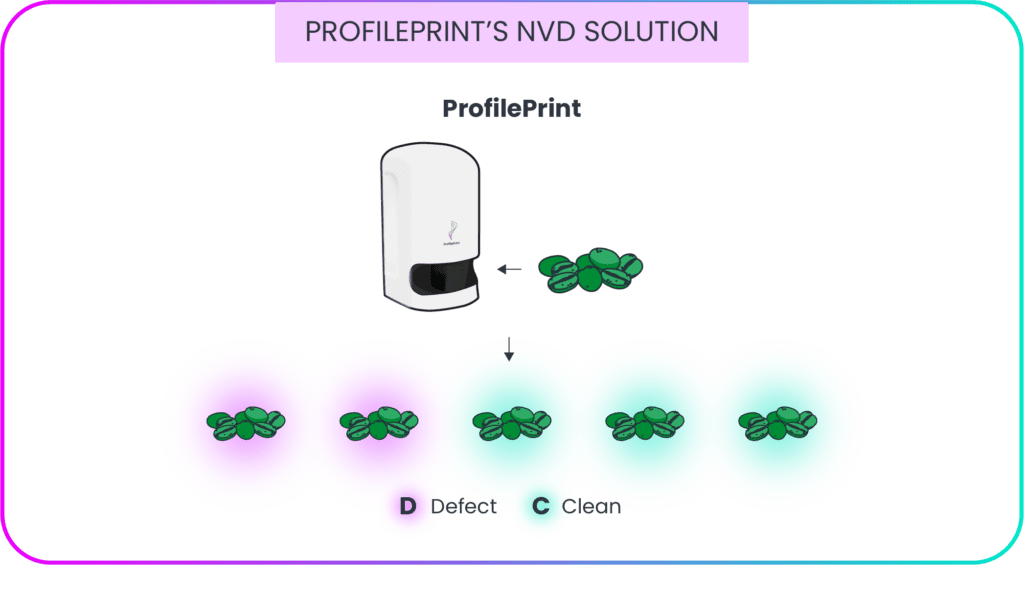
Our NVD solution leverages innovative sample scanning methodologies and advanced machine learning algorithms to pick out defective coffee beans scattered in the specimen dish. The algorithm further judges if a cup of coffee brewed from these beans would be deemed defective by trained cuppers. Let’s explore this in detail.
ProfilePrint’s Cluster Learning for Evaluating Anomalies in Results (CLEAR)
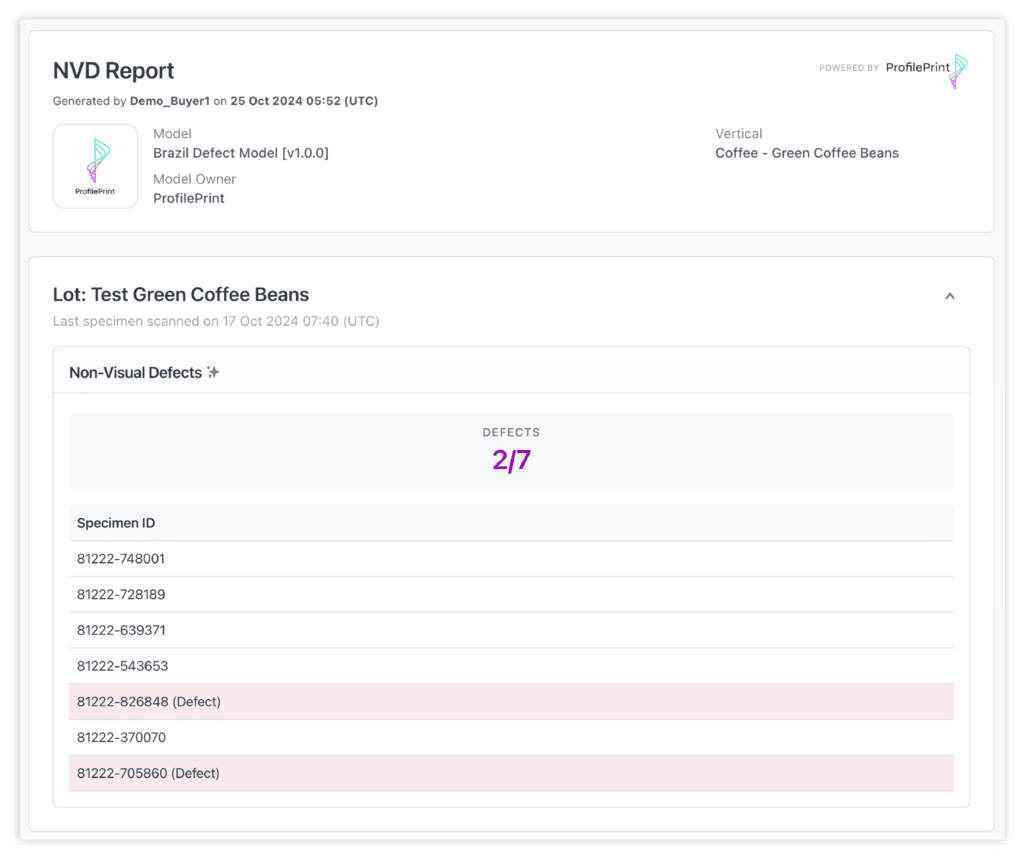
The processed spectra are put through a unique algorithm that groups spectra together based on their similarities. Subsequently, by evaluating the similarities and the number of spectra in each group, the model decides if that dish is considered “Clean” or “Defective”.
Based on our usual protocol of scanning 10 specimens of coffee from a single lot, one is able to infer how many specimens out of 10 are defective, and this can be in turn correlated to the number of cups typically cupped by the coffee tasters, or translated to a certain grade for decision making.
ProfilePrint’s Spatial Scanning Methodology (SpaSM)
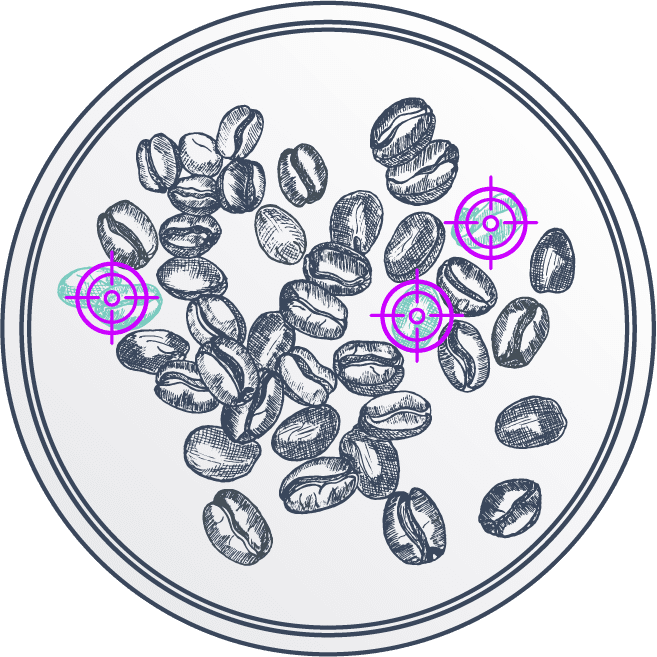
Our analyser leverages the spatial scanning methodology to detect defects in green coffee beans. This analyses the ingredient’s molecular signature, detecting defective beans scattered in a sample by examining their unique chemical composition rather than relying on visual cues or photo recognition. By scanning the specimen dish in a systematic manner, we are able to detect areas in the dish with aberrant fingerprints from the rest of the dish. These unusual fingerprints may correspond to defective coffee beans. Our proprietary data processing pipelines further amplify the differences in the fingerprints to increase the reliability of the evaluation.
CASE STUDY 1
Streamlining Coffee Bean Quality Assessment
CHALLENGE:
A leading Brazilian coffee bean exporter was looking to improve their quality assessment process during peak harvest season. They were looking for a way to quickly identify defective coffee beans.
SOLUTION:
ProfilePrint’s NVD detection solution can be used as a screening tool to distinguish between clean and defective cups. Our client provided a variety of green coffee bean samples, and the model was trained on 10 clean lots and 48 defective lots, covering 4 defect types.
OUTCOME:
Despite the limited sample size, ProfilePrint was able to correctly identify 80% of the actual clean cups as clean, and 83% of the actual cup defects as defects.
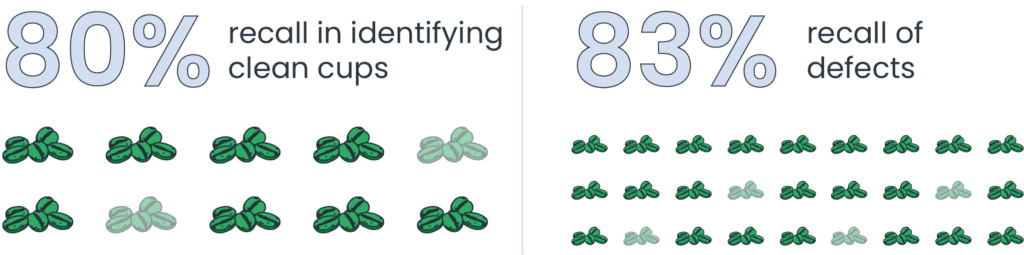
This improvement in efficiency allows the client to focus their team’s resources on the most promising samples, after initial pre-screening by ProfilePrint.
CASE STUDY 2
Tackling Complex Defect Detection
CHALLENGE:
A client in the coffee industry needed a rapid screening tool to save time and resources in their quality control process. Specifically, they aimed to efficiently identify Riado beans, one of the most challenging defects to identify in Brazilian coffee. They are known for their light flavour and distinct medicinal or iodine-like taste, a flavour profile considered unpleasant and can affect the overall quality of the coffee.
SOLUTION:
ProfilePrint developed a customised model to address their unique needs, with a focus on detecting Riado beans. This model was trained with an even smaller dataset of 20 defective lots and 20 clean lots.
OUTCOME:
Despite the complexity of detecting Riado beans and the limited training data, ProfilePrint was able to correctly identify 60% of the actual clean cups as clean, and 95% of the actual cup defects as defects.
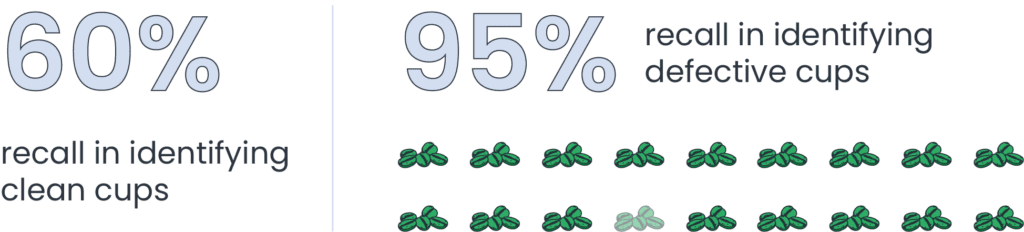
This demonstrates the potential of ProfilePrint’s NVD solution in handling even the most challenging quality control tasks in the coffee industry.
LEARN MORE
ProfilePrint’s early release in 2024 for key clients in Brazil and China has yielded promising results. Stay tuned for updates on our latest development as we pioneer the world’s first commercial A.I. solution for NVD detection in 2025. To learn more about how our NVD solution can elevate your coffee business, contact us for a demonstration.